Retail is an area where innovations are readily tested. Innovative solutions in retail help to automate and speed up processes and improve their quality. However, innovation often becomes surrounded by false narratives.
Mikhail Savitsky, Tech Team Lead of the Goods Checker solution, shared his opinion on computer vision issues he most often faces when communicating with customers.
Table of Сontents
Popular Misconceptions about Computer Vision
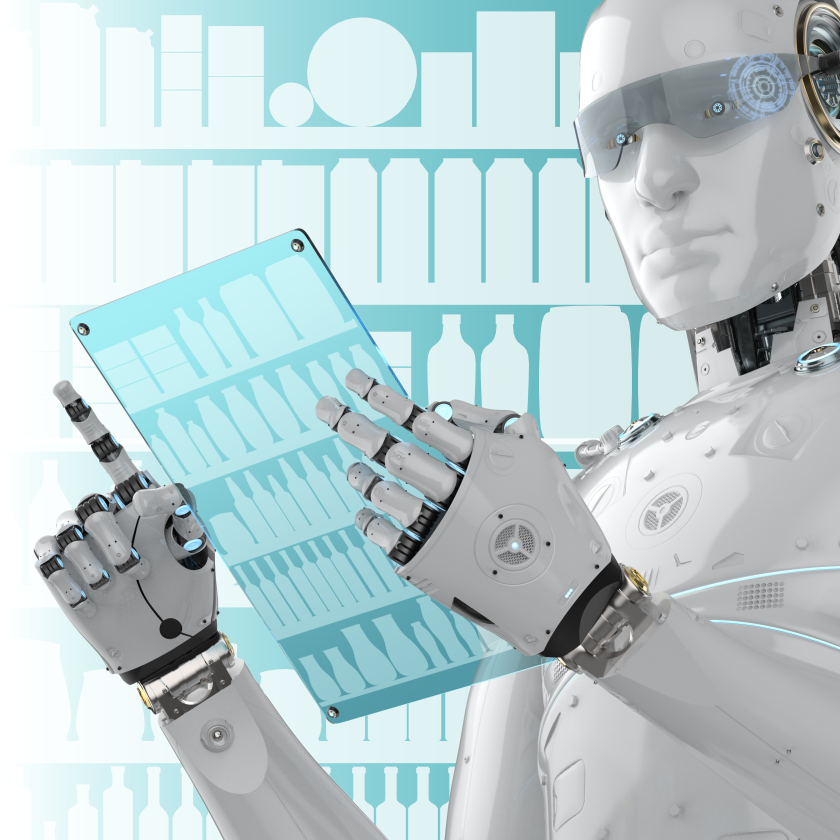
Myth 1. Computer vision requires an expensive smartphone with a good camera.
Some people believe that computer vision works well only if a super-quality camera is used, for example, as that offered by flagship smartphones.
Otherwise, neural networks will not be able to recognize the image correctly.
That’s not true. For the last few years, the common smartphone that most people use has a camera that’s good enough for computer vision to be able to identify the goods in the images.
Myth 2. Humans recognize better than computer vision.
That’s not quite correct. Computer vision always recognizes what a person can see. Sometimes merchandisers send photos where parts of the goods glare or products are placed in a dark corner. Supervisor will not be able to identify such SKUs. But the neural network recognizes goods by analyzing the pixels in the photo.
Myth 3. Neural networks need a lot of photos to recognize a new product.
Neural networks are trained on hundreds and thousands of images. That’s true. But these do not have to be “real-life” photos of actual physical product. To train neural networks, rendering of a 3D product model is often used. Today, one render is sufficient for AI to learn to recognize the product. The process is as follows.
The employee receives a product render. Next, the employee examines photos of the shelves in the store where the product is displayed. This is required to finalize the render and get the image of the product as close to reality as possible. For example, if a product is illuminated with a LED strip, it should be illuminated from the same side on the renderer as well. After such improvements, a set of images (dataset) is obtained, which is used for training the neural network. Upon completion of training, the model’s accuracy is verified with the photos of the actual product.
Long Shelf Problem
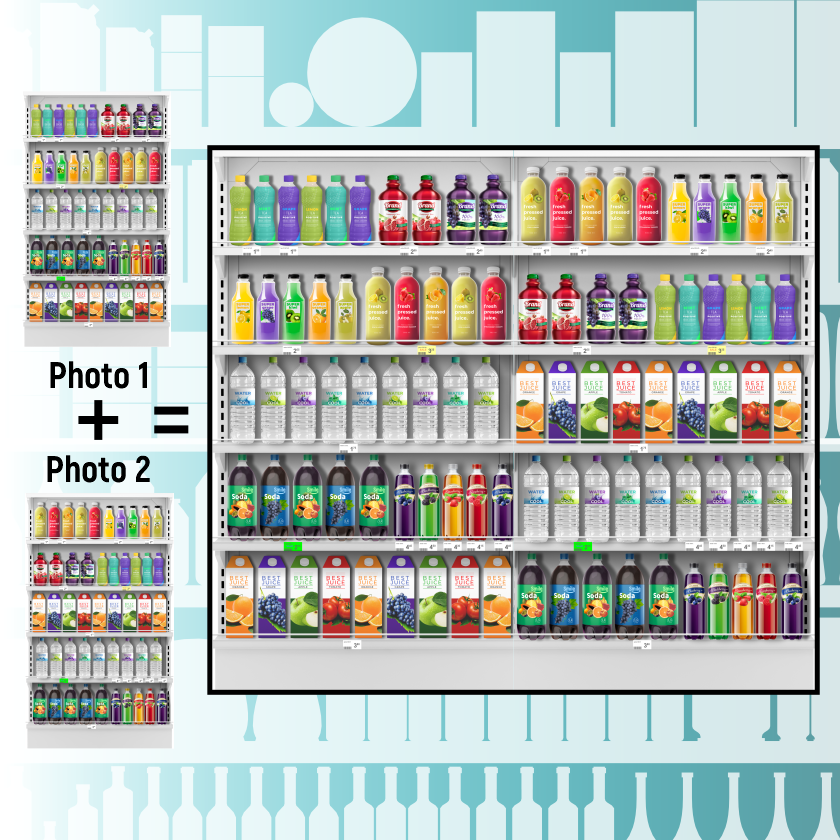
Computer vision also helps to deal with photos of shelves in long, narrow store aisles. It is difficult for a merchandiser to cover the goods on such a rack in one image. Usually long shelves are photographed at an angle, and distant goods are difficult or even impossible to see.
Neural networks can address this issue. The merchandiser takes photos of individual parts of a long rack, and computer vision algorithms merge several photos into one. Merchandiser and supervisor get one long shelf photo and better product recognition.
Computer Vision is an Assistant to an FMCG Company
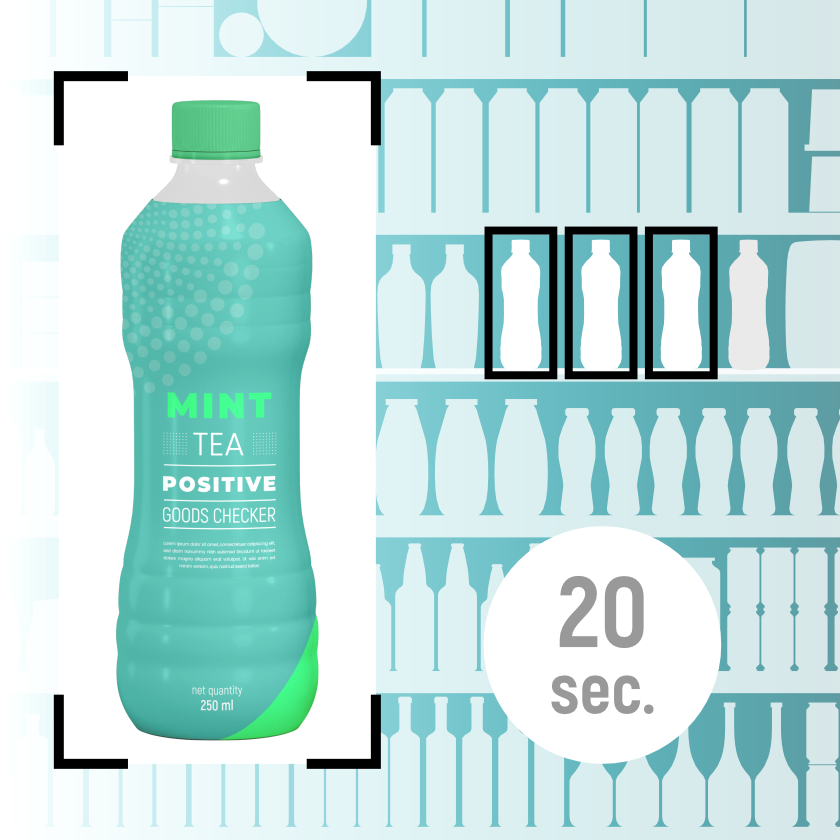
Computer vision recognizes products faster and more accurately than humans. Verification of actual layout against the planogram on a large rack takes 20-30 seconds, a minute at most (depending on the data connectivity speed). But the merchandiser usually takes at least a few minutes to do this. Additionally, by the end of the day, fatigue accumulates, attention is lost and errors occur.
On the other hand, neural networks work accurately and quickly and never get tired. Supervisors and marketers receive information in near real time. This implies that they can quickly respond to any abnormalities, acknowledge or change the marketing strategy thanks to relevant and reliable data.